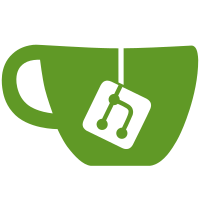
* tests synchro * draft * further draft * affinity ok * proposals okay, need to incorporate in Pose2Sim+tests * will transfer sorting across frames in triangulation in next commit * Lasts tests need to be done but seems to work pretty well * should all work smoothly * update readme * last checks * fixed linting issues * getting tired of being forgetful
724 lines
31 KiB
Python
724 lines
31 KiB
Python
#!/usr/bin/env python
|
|
# -*- coding: utf-8 -*-
|
|
|
|
|
|
'''
|
|
###########################################################################
|
|
## TRACKING OF PERSON OF INTEREST ##
|
|
###########################################################################
|
|
|
|
Openpose detects all people in the field of view.
|
|
- multi_person = false: Which is the one of interest?
|
|
- multi_person = true: How to triangulate the same persons across views?
|
|
How to associate them across time frames? Done in the
|
|
triangulation stage.
|
|
|
|
If multi_person = false, this module tries all possible triangulations of a chosen
|
|
anatomical point, and chooses the person for whom the reprojection error is smallest.
|
|
|
|
If multi_person = true, it computes the distance between epipolar lines (camera to
|
|
keypoint lines) for all persons detected in all views, and selects the best correspondences.
|
|
The computation of the affinity matrix from the distance is inspired from the EasyMocap approach.
|
|
|
|
INPUTS:
|
|
- a calibration file (.toml extension)
|
|
- json files from each camera folders with several detected persons
|
|
- a Config.toml file
|
|
- a skeleton model
|
|
|
|
OUTPUTS:
|
|
- json files for each camera with only one person of interest
|
|
'''
|
|
|
|
|
|
## INIT
|
|
import os
|
|
import glob
|
|
import fnmatch
|
|
import numpy as np
|
|
import json
|
|
import itertools as it
|
|
import toml
|
|
from tqdm import tqdm
|
|
import cv2
|
|
from anytree import RenderTree
|
|
from anytree.importer import DictImporter
|
|
import logging
|
|
|
|
from Pose2Sim.common import retrieve_calib_params, computeP, weighted_triangulation, \
|
|
reprojection, euclidean_distance, natural_sort
|
|
from Pose2Sim.skeletons import *
|
|
|
|
|
|
## AUTHORSHIP INFORMATION
|
|
__author__ = "David Pagnon"
|
|
__copyright__ = "Copyright 2021, Pose2Sim"
|
|
__credits__ = ["David Pagnon"]
|
|
__license__ = "BSD 3-Clause License"
|
|
__version__ = '0.6'
|
|
__maintainer__ = "David Pagnon"
|
|
__email__ = "contact@david-pagnon.com"
|
|
__status__ = "Development"
|
|
|
|
|
|
## FUNCTIONS
|
|
def persons_combinations(json_files_framef):
|
|
'''
|
|
Find all possible combinations of detected persons' ids.
|
|
Person's id when no person detected is set to -1.
|
|
|
|
INPUT:
|
|
- json_files_framef: list of strings
|
|
|
|
OUTPUT:
|
|
- personsIDs_comb: array, list of lists of int
|
|
'''
|
|
|
|
n_cams = len(json_files_framef)
|
|
|
|
# amount of persons detected for each cam
|
|
nb_persons_per_cam = []
|
|
for c in range(n_cams):
|
|
with open(json_files_framef[c], 'r') as js:
|
|
nb_persons_per_cam += [len(json.load(js)['people'])]
|
|
|
|
# persons_combinations
|
|
id_no_detect = [i for i, x in enumerate(nb_persons_per_cam) if x == 0] # ids of cameras that have not detected any person
|
|
nb_persons_per_cam = [x if x != 0 else 1 for x in nb_persons_per_cam] # temporarily replace persons count by 1 when no detection
|
|
range_persons_per_cam = [range(nb_persons_per_cam[c]) for c in range(n_cams)]
|
|
personsIDs_comb = np.array(list(it.product(*range_persons_per_cam)), float) # all possible combinations of persons' ids
|
|
personsIDs_comb[:,id_no_detect] = np.nan # -1 = persons' ids when no person detected
|
|
|
|
return personsIDs_comb
|
|
|
|
|
|
def triangulate_comb(comb, coords, P_all, calib_params, config):
|
|
'''
|
|
Triangulate 2D points and compute reprojection error for a combination of cameras.
|
|
INPUTS:
|
|
- comb: list of ints: combination of persons' ids for each camera
|
|
- coords: array: x, y, likelihood for each camera
|
|
- P_all: list of arrays: projection matrices for each camera
|
|
- calib_params: dict: calibration parameters
|
|
- config: dictionary from Config.toml file
|
|
OUTPUTS:
|
|
- error_comb: float: reprojection error
|
|
- comb: list of ints: combination of persons' ids for each camera
|
|
- Q_comb: array: 3D coordinates of the triangulated point
|
|
'''
|
|
|
|
undistort_points = config.get('triangulation').get('undistort_points')
|
|
likelihood_threshold = config.get('personAssociation').get('likelihood_threshold_association')
|
|
|
|
# Replace likelihood by 0. if under likelihood_threshold
|
|
coords[:,2][coords[:,2] < likelihood_threshold] = 0.
|
|
comb[coords[:,2] == 0.] = np.nan
|
|
|
|
# Filter coords and projection_matrices containing nans
|
|
coords_filt = [coords[i] for i in range(len(comb)) if not np.isnan(comb[i])]
|
|
projection_matrices_filt = [P_all[i] for i in range(len(comb)) if not np.isnan(comb[i])]
|
|
if undistort_points:
|
|
calib_params_R_filt = [calib_params['R'][i] for i in range(len(comb)) if not np.isnan(comb[i])]
|
|
calib_params_T_filt = [calib_params['T'][i] for i in range(len(comb)) if not np.isnan(comb[i])]
|
|
calib_params_K_filt = [calib_params['K'][i] for i in range(len(comb)) if not np.isnan(comb[i])]
|
|
calib_params_dist_filt = [calib_params['dist'][i] for i in range(len(comb)) if not np.isnan(comb[i])]
|
|
|
|
# Triangulate 2D points
|
|
x_files_filt, y_files_filt, likelihood_files_filt = np.array(coords_filt).T
|
|
Q_comb = weighted_triangulation(projection_matrices_filt, x_files_filt, y_files_filt, likelihood_files_filt)
|
|
|
|
# Reprojection
|
|
if undistort_points:
|
|
coords_2D_kpt_calc_filt = [cv2.projectPoints(np.array(Q_comb[:-1]), calib_params_R_filt[i], calib_params_T_filt[i], calib_params_K_filt[i], calib_params_dist_filt[i])[0] for i in range(len(Q_comb))]
|
|
x_calc = [coords_2D_kpt_calc_filt[i][0,0,0] for i in range(len(Q_comb))]
|
|
y_calc = [coords_2D_kpt_calc_filt[i][0,0,1] for i in range(len(Q_comb))]
|
|
else:
|
|
x_calc, y_calc = reprojection(projection_matrices_filt, Q_comb)
|
|
|
|
# Reprojection error
|
|
error_comb_per_cam = []
|
|
for cam in range(len(x_calc)):
|
|
q_file = (x_files_filt[cam], y_files_filt[cam])
|
|
q_calc = (x_calc[cam], y_calc[cam])
|
|
error_comb_per_cam.append( euclidean_distance(q_file, q_calc) )
|
|
error_comb = np.mean(error_comb_per_cam)
|
|
|
|
return error_comb, comb, Q_comb
|
|
|
|
|
|
def best_persons_and_cameras_combination(config, json_files_framef, personsIDs_combinations, projection_matrices, tracked_keypoint_id, calib_params):
|
|
'''
|
|
Chooses the right person among the multiple ones found by
|
|
OpenPose & excludes cameras with wrong 2d-pose estimation.
|
|
|
|
1. triangulate the tracked keypoint for all possible combinations of people,
|
|
2. compute difference between reprojection & original openpose detection,
|
|
3. take combination with smallest error OR all those below the error threshold
|
|
If error is too big, take off one or several of the cameras until err is
|
|
lower than "max_err_px".
|
|
|
|
INPUTS:
|
|
- a Config.toml file
|
|
- json_files_framef: list of strings
|
|
- personsIDs_combinations: array, list of lists of int
|
|
- projection_matrices: list of arrays
|
|
- tracked_keypoint_id: int
|
|
|
|
OUTPUTS:
|
|
- errors_below_thresh: list of float
|
|
- comb_errors_below_thresh: list of arrays of ints
|
|
'''
|
|
|
|
error_threshold_tracking = config.get('personAssociation').get('single_person').get('reproj_error_threshold_association')
|
|
likelihood_threshold = config.get('personAssociation').get('likelihood_threshold_association')
|
|
min_cameras_for_triangulation = config.get('triangulation').get('min_cameras_for_triangulation')
|
|
undistort_points = config.get('triangulation').get('undistort_points')
|
|
|
|
n_cams = len(json_files_framef)
|
|
error_min = np.inf
|
|
nb_cams_off = 0 # cameras will be taken-off until the reprojection error is under threshold
|
|
errors_below_thresh = []
|
|
comb_errors_below_thresh = []
|
|
Q_kpt = []
|
|
while error_min > error_threshold_tracking and n_cams - nb_cams_off >= min_cameras_for_triangulation:
|
|
# Try all persons combinations
|
|
for combination in personsIDs_combinations:
|
|
# Get coords from files
|
|
coords = []
|
|
for index_cam, person_nb in enumerate(combination):
|
|
try:
|
|
js = read_json(json_files_framef[index_cam])
|
|
coords.append(js[int(person_nb)][tracked_keypoint_id*3:tracked_keypoint_id*3+3])
|
|
except:
|
|
coords.append([np.nan, np.nan, np.nan])
|
|
coords = np.array(coords)
|
|
|
|
# undistort points
|
|
if undistort_points:
|
|
points = np.array(coords)[:,None,:2]
|
|
undistorted_points = [cv2.undistortPoints(points[i], calib_params['K'][i], calib_params['dist'][i], None, calib_params['optim_K'][i]) for i in range(n_cams)]
|
|
coords[:,0] = np.array([[u[i][0][0] for i in range(len(u))] for u in undistorted_points]).squeeze()
|
|
coords[:,1] = np.array([[u[i][0][1] for i in range(len(u))] for u in undistorted_points]).squeeze()
|
|
|
|
# For each persons combination, create subsets with "nb_cams_off" cameras excluded
|
|
id_cams_off = list(it.combinations(range(len(combination)), nb_cams_off))
|
|
combinations_with_cams_off = np.array([combination.copy()]*len(id_cams_off))
|
|
for i, id in enumerate(id_cams_off):
|
|
combinations_with_cams_off[i,id] = np.nan
|
|
|
|
# Try all subsets
|
|
error_comb_all, comb_all, Q_comb_all = [], [], []
|
|
for comb in combinations_with_cams_off:
|
|
error_comb, comb, Q_comb = triangulate_comb(comb, coords, projection_matrices, calib_params, config)
|
|
error_comb_all.append(error_comb)
|
|
comb_all.append(comb)
|
|
Q_comb_all.append(Q_comb)
|
|
|
|
error_min = np.nanmin(error_comb_all)
|
|
comb_error_min = [comb_all[np.argmin(error_comb_all)]]
|
|
Q_kpt = [Q_comb_all[np.argmin(error_comb_all)]]
|
|
if error_min < error_threshold_tracking:
|
|
break
|
|
|
|
nb_cams_off += 1
|
|
|
|
return error_min, comb_error_min, Q_kpt
|
|
|
|
|
|
def read_json(js_file):
|
|
'''
|
|
Read OpenPose json file
|
|
'''
|
|
with open(js_file, 'r') as json_f:
|
|
js = json.load(json_f)
|
|
json_data = []
|
|
for people in range(len(js['people'])):
|
|
if len(js['people'][people]['pose_keypoints_2d']) < 3: continue
|
|
else:
|
|
json_data.append(js['people'][people]['pose_keypoints_2d'])
|
|
return json_data
|
|
|
|
|
|
def compute_rays(json_coord, calib_params, cam_id):
|
|
'''
|
|
Plucker coordinates of rays from camera to each joint of a person
|
|
Plucker coordinates: camera to keypoint line direction (size 3)
|
|
moment: origin ^ line (size 3)
|
|
additionally, confidence
|
|
|
|
INPUTS:
|
|
- json_coord: x, y, likelihood for a person seen from a camera (list of 3*joint_nb)
|
|
- calib_params: calibration parameters from retrieve_calib_params('calib.toml')
|
|
- cam_id: camera id (int)
|
|
|
|
OUTPUT:
|
|
- plucker: array. nb joints * (6 plucker coordinates + 1 likelihood)
|
|
'''
|
|
|
|
x = json_coord[0::3]
|
|
y = json_coord[1::3]
|
|
likelihood = json_coord[2::3]
|
|
|
|
inv_K = calib_params['inv_K'][cam_id]
|
|
R_mat = calib_params['R_mat'][cam_id]
|
|
T = calib_params['T'][cam_id]
|
|
|
|
cam_center = -R_mat.T @ T
|
|
plucker = []
|
|
for i in range(len(x)):
|
|
q = np.array([x[i], y[i], 1])
|
|
norm_Q = R_mat.T @ (inv_K @ q -T)
|
|
|
|
line = norm_Q - cam_center
|
|
norm_line = line/np.linalg.norm(line)
|
|
moment = np.cross(cam_center, norm_line)
|
|
plucker.append(np.concatenate([norm_line, moment, [likelihood[i]]]))
|
|
|
|
return np.array(plucker)
|
|
|
|
|
|
def broadcast_line_to_line_distance(p0, p1):
|
|
'''
|
|
Compute the distance between two lines in 3D space.
|
|
|
|
see: https://faculty.sites.iastate.edu/jia/files/inline-files/plucker-coordinates.pdf
|
|
p0 = (l0,m0), p1 = (l1,m1)
|
|
dist = | (l0,m0) * (l1,m1) | / || l0 x l1 ||
|
|
(l0,m0) * (l1,m1) = l0 @ m1 + m0 @ l1 (reciprocal product)
|
|
|
|
No need to divide by the norm of the cross product of the directions, since we
|
|
don't need the actual distance but whether the lines are close to intersecting or not
|
|
=> dist = | (l0,m0) * (l1,m1) |
|
|
|
|
INPUTS:
|
|
- p0: array(nb_persons_detected * 1 * nb_joints * 7 coordinates)
|
|
- p1: array(1 * nb_persons_detected * nb_joints * 7 coordinates)
|
|
|
|
OUTPUT:
|
|
- dist: distances between the two lines (not normalized).
|
|
array(nb_persons_0 * nb_persons_1 * nb_joints)
|
|
'''
|
|
|
|
product = np.sum(p0[..., :3] * p1[..., 3:6], axis=-1) + np.sum(p1[..., :3] * p0[..., 3:6], axis=-1)
|
|
dist = np.abs(product)
|
|
|
|
return dist
|
|
|
|
|
|
def compute_affinity(all_json_data_f, calib_params, cum_persons_per_view, reconstruction_error_threshold=0.1):
|
|
'''
|
|
Compute the affinity between all the people in the different views.
|
|
|
|
The affinity is defined as 1 - distance/max_distance, with distance the
|
|
distance between epipolar lines in each view (reciprocal product of Plucker
|
|
coordinates).
|
|
|
|
Another approach would be to project one epipolar line onto the other camera
|
|
plane and compute the line to point distance, but it is more computationally
|
|
intensive (simple dot product vs. projection and distance calculation).
|
|
|
|
INPUTS:
|
|
- all_json_data_f: list of json data. For frame f, nb_views*nb_persons*(x,y,likelihood)*nb_joints
|
|
- calib_params: calibration parameters from retrieve_calib_params('calib.toml')
|
|
- cum_persons_per_view: cumulative number of persons per view
|
|
- reconstruction_error_threshold: maximum distance between epipolar lines to consider a match
|
|
|
|
OUTPUT:
|
|
- affinity: affinity matrix between all the people in the different views.
|
|
(nb_views*nb_persons_per_view * nb_views*nb_persons_per_view)
|
|
'''
|
|
|
|
# Compute plucker coordinates for all keypoints for each person in each view
|
|
# pluckers_f: dims=(camera, person, joint, 7 coordinates)
|
|
pluckers_f = []
|
|
for cam_id, json_cam in enumerate(all_json_data_f):
|
|
pluckers = []
|
|
for json_coord in json_cam:
|
|
plucker = compute_rays(json_coord, calib_params, cam_id) # LIMIT TO 15 JOINTS? json_coord[:15*3]
|
|
pluckers.append(plucker)
|
|
pluckers = np.array(pluckers)
|
|
pluckers_f.append(pluckers)
|
|
|
|
# Compute affinity matrix
|
|
distance = np.zeros((cum_persons_per_view[-1], cum_persons_per_view[-1])) + 2*reconstruction_error_threshold
|
|
for compared_cam0, compared_cam1 in it.combinations(range(len(all_json_data_f)), 2):
|
|
# skip when no detection for a camera
|
|
if cum_persons_per_view[compared_cam0] == cum_persons_per_view[compared_cam0+1] \
|
|
or cum_persons_per_view[compared_cam1] == cum_persons_per_view[compared_cam1 +1]:
|
|
continue
|
|
|
|
# compute distance
|
|
p0 = pluckers_f[compared_cam0][:,None] # add coordinate on second dimension
|
|
p1 = pluckers_f[compared_cam1][None,:] # add coordinate on first dimension
|
|
dist = broadcast_line_to_line_distance(p0, p1)
|
|
likelihood = np.sqrt(p0[..., -1] * p1[..., -1])
|
|
mean_weighted_dist = np.sum(dist*likelihood, axis=-1)/(1e-5 + likelihood.sum(axis=-1)) # array(nb_persons_0 * nb_persons_1)
|
|
|
|
# populate distance matrix
|
|
distance[cum_persons_per_view[compared_cam0]:cum_persons_per_view[compared_cam0+1], \
|
|
cum_persons_per_view[compared_cam1]:cum_persons_per_view[compared_cam1+1]] \
|
|
= mean_weighted_dist
|
|
distance[cum_persons_per_view[compared_cam1]:cum_persons_per_view[compared_cam1+1], \
|
|
cum_persons_per_view[compared_cam0]:cum_persons_per_view[compared_cam0+1]] \
|
|
= mean_weighted_dist.T
|
|
|
|
# compute affinity matrix and clamp it to zero when distance > reconstruction_error_threshold
|
|
distance[distance > reconstruction_error_threshold] = reconstruction_error_threshold
|
|
affinity = 1 - distance / reconstruction_error_threshold
|
|
|
|
return affinity
|
|
|
|
|
|
def circular_constraint(cum_persons_per_view):
|
|
'''
|
|
A person can be matched only with themselves in the same view, and with any
|
|
person from other views
|
|
|
|
INPUT:
|
|
- cum_persons_per_view: cumulative number of persons per view
|
|
|
|
OUTPUT:
|
|
- circ_constraint: circular constraint matrix
|
|
'''
|
|
|
|
circ_constraint = np.identity(cum_persons_per_view[-1])
|
|
for i in range(len(cum_persons_per_view)-1):
|
|
circ_constraint[cum_persons_per_view[i]:cum_persons_per_view[i+1], cum_persons_per_view[i+1]:cum_persons_per_view[-1]] = 1
|
|
circ_constraint[cum_persons_per_view[i+1]:cum_persons_per_view[-1], cum_persons_per_view[i]:cum_persons_per_view[i+1]] = 1
|
|
|
|
return circ_constraint
|
|
|
|
|
|
def SVT(matrix, threshold):
|
|
'''
|
|
Find a low-rank approximation of the matrix using Singular Value Thresholding.
|
|
|
|
INPUTS:
|
|
- matrix: matrix to decompose
|
|
- threshold: threshold for singular values
|
|
|
|
OUTPUT:
|
|
- matrix_thresh: low-rank approximation of the matrix
|
|
'''
|
|
|
|
U, s, Vt = np.linalg.svd(matrix) # decompose matrix
|
|
s_thresh = np.maximum(s - threshold, 0) # set smallest singular values to zero
|
|
matrix_thresh = U @ np.diag(s_thresh) @ Vt # recompose matrix
|
|
|
|
return matrix_thresh
|
|
|
|
|
|
def matchSVT(affinity, cum_persons_per_view, circ_constraint, max_iter = 20, w_rank = 50, tol = 1e-4, w_sparse=0.1):
|
|
'''
|
|
Find low-rank approximation of 'affinity' while satisfying the circular constraint.
|
|
|
|
INPUTS:
|
|
- affinity: affinity matrix between all the people in the different views
|
|
- cum_persons_per_view: cumulative number of persons per view
|
|
- circ_constraint: circular constraint matrix
|
|
- max_iter: maximum number of iterations
|
|
- w_rank: threshold for singular values
|
|
- tol: tolerance for convergence
|
|
- w_sparse: regularization parameter
|
|
|
|
OUTPUT:
|
|
- new_aff: low-rank approximation of the affinity matrix
|
|
'''
|
|
|
|
new_aff = affinity.copy()
|
|
N = new_aff.shape[0]
|
|
index_diag = np.arange(N)
|
|
new_aff[index_diag, index_diag] = 0.
|
|
# new_aff = (new_aff + new_aff.T)/2 # symmetric by construction
|
|
|
|
Y = np.zeros_like(new_aff) # Initial deviation matrix / residual ()
|
|
W = w_sparse - new_aff # Initial sparse matrix / regularization (prevent overfitting)
|
|
mu = 64 # initial step size
|
|
|
|
for iter in range(max_iter):
|
|
new_aff0 = new_aff.copy()
|
|
|
|
Q = new_aff + Y*1.0/mu
|
|
Q = SVT(Q,w_rank/mu)
|
|
new_aff = Q - (W + Y)/mu
|
|
|
|
# Project X onto dimGroups
|
|
for i in range(len(cum_persons_per_view) - 1):
|
|
ind1, ind2 = cum_persons_per_view[i], cum_persons_per_view[i + 1]
|
|
new_aff[ind1:ind2, ind1:ind2] = 0
|
|
|
|
# Reset diagonal elements to one and ensure X is within valid range [0, 1]
|
|
new_aff[index_diag, index_diag] = 1.
|
|
new_aff[new_aff < 0] = 0
|
|
new_aff[new_aff > 1] = 1
|
|
|
|
# Enforce circular constraint
|
|
new_aff = new_aff * circ_constraint
|
|
new_aff = (new_aff + new_aff.T) / 2 # kept just in case X loses its symmetry during optimization
|
|
Y = Y + mu * (new_aff - Q)
|
|
|
|
# Compute convergence criteria: break if new_aff is close enough to Q and no evolution anymore
|
|
pRes = np.linalg.norm(new_aff - Q) / N # primal residual (diff between new_aff and SVT result)
|
|
dRes = mu * np.linalg.norm(new_aff - new_aff0) / N # dual residual (diff between new_aff and previous new_aff)
|
|
if pRes < tol and dRes < tol:
|
|
break
|
|
if pRes > 10 * dRes: mu = 2 * mu
|
|
elif dRes > 10 * pRes: mu = mu / 2
|
|
|
|
iter +=1
|
|
|
|
return new_aff
|
|
|
|
|
|
def person_index_per_cam(affinity, cum_persons_per_view, min_cameras_for_triangulation):
|
|
'''
|
|
For each detected person, gives their index for each camera
|
|
|
|
INPUTS:
|
|
- affinity: affinity matrix between all the people in the different views
|
|
- min_cameras_for_triangulation: exclude proposals if less than N cameras see them
|
|
|
|
OUTPUT:
|
|
- proposals: 2D array: n_persons * n_cams
|
|
'''
|
|
|
|
# index of the max affinity for each group (-1 if no detection)
|
|
proposals = []
|
|
for row in range(affinity.shape[0]):
|
|
proposal_row = []
|
|
for cam in range(len(cum_persons_per_view)-1):
|
|
id_persons_per_view = affinity[row, cum_persons_per_view[cam]:cum_persons_per_view[cam+1]]
|
|
proposal_row += [np.argmax(id_persons_per_view) if (len(id_persons_per_view)>0 and max(id_persons_per_view)>0) else -1]
|
|
proposals.append(proposal_row)
|
|
proposals = np.array(proposals, dtype=float)
|
|
|
|
# remove duplicates and order
|
|
proposals, nb_detections = np.unique(proposals, axis=0, return_counts=True)
|
|
proposals = proposals[np.argsort(nb_detections)[::-1]]
|
|
|
|
# remove row if any value is the same in previous rows at same index (nan!=nan so nan ignored)
|
|
proposals[proposals==-1] = np.nan
|
|
mask = np.ones(proposals.shape[0], dtype=bool)
|
|
for i in range(1, len(proposals)):
|
|
mask[i] = ~np.any(proposals[i] == proposals[:i], axis=0).any()
|
|
proposals = proposals[mask]
|
|
|
|
# remove identifications if less than N cameras see them
|
|
nb_cams_per_person = [np.count_nonzero(~np.isnan(p)) for p in proposals]
|
|
proposals = np.array([p for (n,p) in zip(nb_cams_per_person, proposals) if n >= min_cameras_for_triangulation])
|
|
|
|
return proposals
|
|
|
|
|
|
def rewrite_json_files(json_tracked_files_f, json_files_f, proposals, n_cams):
|
|
'''
|
|
Write new json files with correct association of people across cameras.
|
|
|
|
INPUTS:
|
|
- json_tracked_files_f: list of strings: json files to write
|
|
- json_files_f: list of strings: json files to read
|
|
- proposals: 2D array: n_persons * n_cams
|
|
- n_cams: int: number of cameras
|
|
|
|
OUTPUT:
|
|
- json files with correct association of people across cameras
|
|
'''
|
|
|
|
for cam in range(n_cams):
|
|
with open(json_tracked_files_f[cam], 'w') as json_tracked_f:
|
|
with open(json_files_f[cam], 'r') as json_f:
|
|
js = json.load(json_f)
|
|
js_new = js.copy()
|
|
js_new['people'] = []
|
|
for new_comb in proposals:
|
|
if not np.isnan(new_comb[cam]):
|
|
js_new['people'] += [js['people'][int(new_comb[cam])]]
|
|
else:
|
|
js_new['people'] += [{}]
|
|
json_tracked_f.write(json.dumps(js_new))
|
|
|
|
|
|
def recap_tracking(config, error=0, nb_cams_excluded=0):
|
|
'''
|
|
Print a message giving statistics on reprojection errors (in pixel and in m)
|
|
as well as the number of cameras that had to be excluded to reach threshold
|
|
conditions. Also stored in User/logs.txt.
|
|
|
|
INPUT:
|
|
- a Config.toml file
|
|
- error: dataframe
|
|
- nb_cams_excluded: dataframe
|
|
|
|
OUTPUT:
|
|
- Message in console
|
|
'''
|
|
|
|
# Read config
|
|
project_dir = config.get('project').get('project_dir')
|
|
session_dir = os.path.realpath(os.path.join(project_dir, '..', '..'))
|
|
multi_person = config.get('project').get('multi_person')
|
|
likelihood_threshold_association = config.get('personAssociation').get('likelihood_threshold_association')
|
|
tracked_keypoint = config.get('personAssociation').get('single_person').get('tracked_keypoint')
|
|
error_threshold_tracking = config.get('personAssociation').get('single_person').get('reproj_error_threshold_association')
|
|
reconstruction_error_threshold = config.get('personAssociation').get('multi_person').get('reconstruction_error_threshold')
|
|
min_affinity = config.get('personAssociation').get('multi_person').get('min_affinity')
|
|
poseTracked_dir = os.path.join(project_dir, 'pose-associated')
|
|
calib_dir = [os.path.join(session_dir, c) for c in os.listdir(session_dir) if 'calib' in c.lower()][0]
|
|
calib_file = glob.glob(os.path.join(calib_dir, '*.toml'))[0] # lastly created calibration file
|
|
|
|
if not multi_person:
|
|
logging.info('\nSingle-person analysis selected.')
|
|
# Error
|
|
mean_error_px = np.around(np.mean(error), decimals=1)
|
|
|
|
calib = toml.load(calib_file)
|
|
calib_cam1 = calib[list(calib.keys())[0]]
|
|
fm = calib_cam1['matrix'][0][0]
|
|
Dm = euclidean_distance(calib_cam1['translation'], [0,0,0])
|
|
mean_error_mm = np.around(mean_error_px * Dm / fm * 1000, decimals=1)
|
|
|
|
# Excluded cameras
|
|
mean_cam_off_count = np.around(np.mean(nb_cams_excluded), decimals=2)
|
|
|
|
# Recap
|
|
logging.info(f'\n--> Mean reprojection error for {tracked_keypoint} point on all frames is {mean_error_px} px, which roughly corresponds to {mean_error_mm} mm. ')
|
|
logging.info(f'--> In average, {mean_cam_off_count} cameras had to be excluded to reach the demanded {error_threshold_tracking} px error threshold after excluding points with likelihood below {likelihood_threshold_association}.')
|
|
|
|
else:
|
|
logging.info('\nMulti-person analysis selected.')
|
|
logging.info(f'\n--> A person was reconstructed if the lines from cameras to their keypoints intersected within {reconstruction_error_threshold} m and if the calculated affinity stayed below {min_affinity} after excluding points with likelihood below {likelihood_threshold_association}.')
|
|
logging.info(f'--> Beware that people were sorted across cameras, but not across frames. This will be done in the triangulation stage.')
|
|
|
|
logging.info(f'\nTracked json files are stored in {os.path.realpath(poseTracked_dir)}.')
|
|
|
|
|
|
def track_2d_all(config):
|
|
'''
|
|
For each frame,
|
|
- Find all possible combinations of detected persons
|
|
- Triangulate 'tracked_keypoint' for all combinations
|
|
- Reproject the point on all cameras
|
|
- Take combination with smallest reprojection error
|
|
- Write json file with only one detected person
|
|
Print recap message
|
|
|
|
INPUTS:
|
|
- a calibration file (.toml extension)
|
|
- json files from each camera folders with several detected persons
|
|
- a Config.toml file
|
|
- a skeleton model
|
|
|
|
OUTPUTS:
|
|
- json files for each camera with only one person of interest
|
|
'''
|
|
|
|
# Read config
|
|
project_dir = config.get('project').get('project_dir')
|
|
session_dir = os.path.realpath(os.path.join(project_dir, '..', '..'))
|
|
multi_person = config.get('project').get('multi_person')
|
|
pose_model = config.get('pose').get('pose_model')
|
|
tracked_keypoint = config.get('personAssociation').get('single_person').get('tracked_keypoint')
|
|
likelihood_threshold = config.get('personAssociation').get('likelihood_threshold_association')
|
|
min_cameras_for_triangulation = config.get('triangulation').get('min_cameras_for_triangulation')
|
|
reconstruction_error_threshold = config.get('personAssociation').get('multi_person').get('reconstruction_error_threshold')
|
|
min_affinity = config.get('personAssociation').get('multi_person').get('min_affinity')
|
|
frame_range = config.get('project').get('frame_range')
|
|
undistort_points = config.get('triangulation').get('undistort_points')
|
|
|
|
calib_dir = [os.path.join(session_dir, c) for c in os.listdir(session_dir) if 'calib' in c.lower() ][0]
|
|
try:
|
|
calib_file = glob.glob(os.path.join(calib_dir, '*.toml'))[0] # lastly created calibration file
|
|
except:
|
|
raise Exception(f'No .toml calibration file found in the {calib_dir}.')
|
|
pose_dir = os.path.join(project_dir, 'pose')
|
|
poseTracked_dir = os.path.join(project_dir, 'pose-associated')
|
|
|
|
if multi_person:
|
|
logging.info('\nMulti-person analysis selected. Note that you can set this option to false if you only need the main person in the scene.')
|
|
else:
|
|
logging.info('\nSingle-person analysis selected.')
|
|
|
|
# projection matrix from toml calibration file
|
|
P_all = computeP(calib_file, undistort=undistort_points)
|
|
calib_params = retrieve_calib_params(calib_file)
|
|
|
|
# selection of tracked keypoint id
|
|
try: # from skeletons.py
|
|
model = eval(pose_model)
|
|
except:
|
|
try: # from Config.toml
|
|
model = DictImporter().import_(config.get('pose').get(pose_model))
|
|
if model.id == 'None':
|
|
model.id = None
|
|
except:
|
|
raise NameError('Model not found in skeletons.py nor in Config.toml')
|
|
tracked_keypoint_id = [node.id for _, _, node in RenderTree(model) if node.name==tracked_keypoint][0]
|
|
|
|
# 2d-pose files selection
|
|
pose_listdirs_names = next(os.walk(pose_dir))[1]
|
|
pose_listdirs_names = natural_sort(pose_listdirs_names)
|
|
json_dirs_names = [k for k in pose_listdirs_names if 'json' in k]
|
|
json_files_names = [fnmatch.filter(os.listdir(os.path.join(pose_dir, js_dir)), '*.json') for js_dir in json_dirs_names]
|
|
json_files_names = [natural_sort(j) for j in json_files_names]
|
|
json_files = [[os.path.join(pose_dir, j_dir, j_file) for j_file in json_files_names[j]] for j, j_dir in enumerate(json_dirs_names)]
|
|
|
|
# 2d-pose-associated files creation
|
|
if not os.path.exists(poseTracked_dir): os.mkdir(poseTracked_dir)
|
|
try: [os.mkdir(os.path.join(poseTracked_dir,k)) for k in json_dirs_names]
|
|
except: pass
|
|
json_tracked_files = [[os.path.join(poseTracked_dir, j_dir, j_file) for j_file in json_files_names[j]] for j, j_dir in enumerate(json_dirs_names)]
|
|
|
|
f_range = [[min([len(j) for j in json_files])] if frame_range==[] else frame_range][0]
|
|
n_cams = len(json_dirs_names)
|
|
error_min_tot, cameras_off_tot = [], []
|
|
|
|
# Check that camera number is consistent between calibration file and pose folders
|
|
if n_cams != len(P_all):
|
|
raise Exception(f'Error: The number of cameras is not consistent:\
|
|
Found {len(P_all)} cameras in the calibration file,\
|
|
and {n_cams} cameras based on the number of pose folders.')
|
|
|
|
Q_kpt = [np.array([0., 0., 0., 1.])]
|
|
for f in tqdm(range(*f_range)):
|
|
# print(f'\nFrame {f}:')
|
|
json_files_f = [json_files[c][f] for c in range(n_cams)]
|
|
json_tracked_files_f = [json_tracked_files[c][f] for c in range(n_cams)]
|
|
Q_kpt_old = Q_kpt
|
|
|
|
if not multi_person:
|
|
# all possible combinations of persons
|
|
personsIDs_comb = persons_combinations(json_files_f)
|
|
|
|
# choose persons of interest and exclude cameras with bad pose estimation
|
|
error_proposals, proposals, Q_kpt = best_persons_and_cameras_combination(config, json_files_f, personsIDs_comb, P_all, tracked_keypoint_id, calib_params)
|
|
|
|
error_min_tot.append(np.mean(error_proposals))
|
|
cameras_off_count = np.count_nonzero([np.isnan(comb) for comb in proposals]) / len(proposals)
|
|
cameras_off_tot.append(cameras_off_count)
|
|
|
|
else:
|
|
# read data
|
|
all_json_data_f = []
|
|
for js_file in json_files_f:
|
|
all_json_data_f.append(read_json(js_file))
|
|
#TODO: remove people with average likelihood < 0.3, no full torso, less than 12 joints... (cf filter2d in dataset/base.py L498)
|
|
|
|
# obtain proposals after computing affinity between all the people in the different views
|
|
persons_per_view = [0] + [len(j) for j in all_json_data_f]
|
|
cum_persons_per_view = np.cumsum(persons_per_view)
|
|
affinity = compute_affinity(all_json_data_f, calib_params, cum_persons_per_view, reconstruction_error_threshold=reconstruction_error_threshold)
|
|
circ_constraint = circular_constraint(cum_persons_per_view)
|
|
affinity = affinity * circ_constraint
|
|
#TODO: affinity without hand, face, feet (cf ray.py L31)
|
|
affinity = matchSVT(affinity, cum_persons_per_view, circ_constraint, max_iter = 20, w_rank = 50, tol = 1e-4, w_sparse=0.1)
|
|
affinity[affinity<min_affinity] = 0
|
|
proposals = person_index_per_cam(affinity, cum_persons_per_view, min_cameras_for_triangulation)
|
|
|
|
# rewrite json files with a single or multiple persons of interest
|
|
rewrite_json_files(json_tracked_files_f, json_files_f, proposals, n_cams)
|
|
|
|
|
|
# recap message
|
|
recap_tracking(config, error_min_tot, cameras_off_tot)
|
|
|